Performance Prediction for Gas Cylinder Steel
Performance Prediction for Gas Cylinder Steel |
GOST 949-73 Steel Cylinders for Gases |
GOST 949-73 TU 14-3R-10-94
The welding steel of gas cylinder is high quality carbon constructional hot rolling steel which is used to make liquefied petroleum vessel, liquid-acetylene vessel, oxygen vessel and liquid chlorine cylinder. Every year there are about ten million cylinders produced in our country, which need about four hundred kilotons gas cylinder steel. At present, the welding steel of gas cylinder is mostly made in Baosteel Co. and Wuhan iron and steel Co.
China stainless steel products enjoys good quality, but still has some disadvantages in mechanical properties compared with the same foreign products, such as:
(1)There is almost no difference in average mechanical properties between Baosteel’s and NKK’s products, but there is big difference in the discrete degree. The comparison of two companies’ gas cylinder steel performances is described in table 2.
(2)Consumers’ demands for mechanical properties become higher and higher. Moreover, different consumers have various demands even for the same product. So producers are requested to adjust process control parameters at any time to meet the consumers’ needs. However, Baosteel is not able to do so at present.
(3) Steel making and hot rolling are two technological processes involved in gas cylinder steel production. Its composition is controlled in steel making process, while F.T. and C.T. of strip steels are controlled in hot rolling process. The comparison of control quality of gas cylinder steel between Baosteel and NKK is described in table 3, which shows that Baosteel’s control level of chemical compositions is low.
To meet the market demand, in 1993, Baosteel developed welding steel special for gas cylinder steel (B440HP). Its chemical composition and mechanical properties are described in table 1.

Control fact of F.T. and C.T. of Baosteel’s cylinder steel B440HP in Nov. 2002 is described in table 4, which indicates control precision of temperature also needs to be improved.

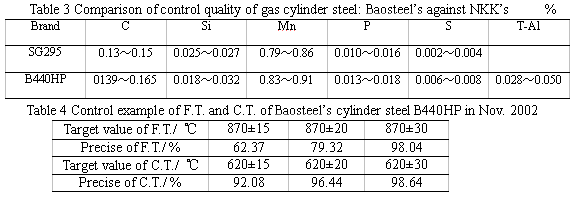
Artificial neural network (abbr. ANN) is a new approach to deal with those problems. ANN is a discriminating way of artificial intelligence model, which resembles brain-neuron knowledge transferring. Its characteristics such as self-organizing, self-learning, and self-adapting enable it to forecast and control nonlinearity system and unknown-model system. This article is to study this system to realize the automatic performance prediction of hot-rolled products. According to consumer needs, we neatly devise the optimal process control parameters to make up the deficiency of steel making process, and predict gas cylinder steel performance by BP, cutting down processing-loss in the course of sampling, improving production yield.
2 The basis of ANN
ANN is a system that consists a great deal of simple processing-units, which resembles the configuration and function of brain-neuron. Due to the increasing high rolling precision, it is impossible to meet the need only depending on some traditional automatic control ways. Because of the unique advantages compared to other automatic control ways, ANN is applied more and more in steel rolling field.
Backward transfer neuron network is one of the widely studied networks, shown in Fig. 1. It includes input layer, hidden layer and output layer. The hidden layer can be single or multiple layers. The neuron on every layer is called node or unit.
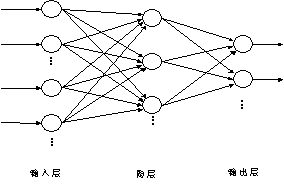
Fig.1 BP network Structure
The activity of input unit represents the initial information inputted to the network. Every hidden unit’s activity is decided by the activity of input unit and the connecting weight between input units. The output unit’s activity is also decided by the activity of hidden unit and the connecting weight between output units. The information transmission above-mentioned is from input unit to hidden unit, then to output unit.
Error backward process algorithm (BP algorithm) realizes the mapping relationship from input to output through a process minimizing cost function. Generally, cost function is defined as error square sum of expected output and practical output of output layer units in input mode.
The computer realizing process of BP algorithm is described as the following:
(1) Initializing, giving all weight value a random arbitrary small value.
(2)Giving training data set,providing input vector Opi and expected output Opj;
(3)Calculating practical output Opj,described in formula (1):

in the formula Wij is a connecting weight from neural I to neural j;Opi is a current input value of neural j;Opj is an output value.
f function is Sigmoid function, described in formula (2):
in the formula, x is independent variable; θ is constant.
(4)Adjusting weight, according to the error backward transferring direction, and from output node back to hidden layer, adjusting weight by formula (3).
in the formula, η is the gain greater than zero; δpj is error of node j; t is hidden layer number. δpj is respectively calculated by formula (4) according to the different status of node j:
in the formula, Tpj is perfect output; δpk is the error of node k; w is the connecting weight from neuron k to neuron j.
Adding momentum item, adjusting weight formula (3) to the following:

in the formula, α is inertia coefficient, 0<α<1.
Repeatedly calculating from formula (5) back to formula (2), until the error meets the demand.
The essence of BP algorithm is a learning method changing a set of sample I/O into non-linear optimized and calculated weight by iteration through gradient algorithm. It has been proved that three-layer neuron network with Sigmoid non-linear function can approach to any continuous function with any precision, which is quite close to the I/O characters of human neuron and has good biotic performances. But BP algorithm still has some inefficiencies, such as low learning efficiency and slow converging rate because of too many iteration algorithms, so in order to speed up convergence and prevent vibration, two method can be used as the following:
(1) Changing value step η: let η gradually decrease with learning processing, viz. Larger η at the beginning can speed up learning, while smaller η can prevent weight coefficient from repeatedly vibrating when approaching the optimal point.
(2) Inertia adjusting: make each adjusting value of weight factor closely relate with the prior weight factor adjusting, creating ‘inertia performance’, then weight adjusting factor can be described in formula (6):

in the formula, n is learning times.
The larger α is, the larger inertia of factor adjusting is, viz. each adjusting value of factor is more closely related with the prior adjusting value.
3 Forecasting gas cylinder steel mechanical property with neuron networks
We train the known sample with the self-learning function of ANN in order to build the forecasting model of gas cylinder steel mechanical property. For selecting a proper forecasting model, firstly we must decide how to select learning-algorithm, learning-parameter, training sample number and data normalizing in order to guarantee good model performance. Secondly, we must discuss the factors influencing the properties of gas cylinder steel and research on every factor influencing feed-forward neuron network’s learning parameters to instruct the buildup of proper forecasting model.
3.1 Defining BP network I/O unit number
Based on the study of Baosteel’s gas cylinder steel, we can conclude the following results from the statistics and analysis of production data:
(1) The most important factor influencing gas cylinder steel mechanical property, steel-making process still has insufficiency. The big fluctuation of metallurgical compositions results in unstable mechanical properties.
(2) Hot rolling process parameters still need to be optimized. The control precision of FT and CT is still not enough.
The key chemical compositions and process parameters of gas cylinder steel B440HP respectively are the content of C, P, S, Mn, Si and RT4(rough rolling temperature), FT (finishing temperature), CT(coiling temperature), which are the input variables in research. Because the thickness of gas cylinder steel produced by Baosteel is all 3.0 mm, the degree of reduction isn’t input variable. The key standards of gas cylinder steel’s mechanical properties include yield strength, tensile strength and percentage extension, so the output variables are settled as σs, σb, δ. Then the input unit number of BP network is 8 and output unit number is 3.
3.2 Researching on BP neuron network’s learning parameter
3.2.1 Sample data selecting and normalizing
The training sample data for experiment all come from Baosteel. The key chemical compositions and process parameters are the content of C, P, S, Mn, Si and RT4(rough rolling temperature), FT (finishing temperature), CT(coiling temperature). And the key data of mechanical properties include yield strength(σ ) , tensile strength(σ ) and percentage extension(δ). As the net output, all data must be normalized. The sample data range is described in table 5:

The map relationship between input and output in feed-forward neuron network is realized through training sample data. Therefore, selecting proper sample is the most important factor influencing model performance. In order to guarantee good performance of building model, firstly, we must select representative sample, secondly, we must define proper number of sample. Insufficient sample cannot cover every status of the modeled object. Under this condition, the model is not representative, not strong, or unable to anti-disturb. On the contrary, overmany samples will result in long learning time.
Applying neuron network in manufacturing, assuring convergence fast and proper, and reflecting the project’s essence, selecting sample data is necessary. We select data by following steps:
(1) 7125 groups of data are drawn from Baosteel 2050 hot-rolling management computer, among which, illogical data are deleted, and 6778 groups of data are left.
(2) For reducing the model’s calculating job, we delete the repeated data with orthogonal experiment concept, and keep 778 groups of data.
(3) We use linear changing method to normalize the data, viz. (X-X )/(X - X ), limiting the sample in the range of 0~1, then those larger inputs are located on high gradient field of neuron network function.
3.2.2 Defining the learning parameter
BP algorithm’s learning parameter include value step η and inertial coefficient α. Under the same network structure and learning precision, sample data are trained by different combinations of α and η. Experiment condition is as the following: network structure is 8-12-3, learning precision is 0.05, sample number is 778, and samples are supplied randomly.
The result indicates that learning parameter puts quite big influence on model’s converging speed. Learning step decides the learning speed. With η gradually increasing, network converging rate is speeding up, and when η is smaller or α is smaller, the effect is more obvious. When η ranges in 0.6~0.8, network converging rate doesn’t speed up greatly for middle α. When η equals 0.9, converging rate isn’t stable even decreases a little bit. At the same time, with η increasing, α is larger, and vibration occurs, so that converging rate decreases or convergence even fails, and converging stability also decreases. To forecast gas cylinder steel performance, considering both network’s converging rate and converging stability, we settle the learning parameter’s best range as η=0.4~0.8,α=0.4~0.6.
When high learning precision is required, we can change value step η, viz. learning with larger η at the beginning in order to speed up convergence, and use smaller η at the stable point to get high converging precision.
3.2.3 Defining network structure parameters
Feed-forward neuron network structure parameters include input layer unit number, output layer unit number, hidden layer number and hidden layer unit number. The former two are commonly defined according to practice, while the definition of hidden layer number and hidden layer unit number is crucial.
To decide the hidden layer number and hidden layer unit number’s influences on model performance, we select different hidden layer number and hidden layer unit number to train the network under the same condition. There are 778 groups of experiments conducted. The experiment conditions and part results are described in table 6.
The experiment results show:
(1) Single hidden layer network’s learning times and converging time gradually decrease with the hidden layer units’ increasing. The increase of hidden layer units results in sharp increase of basic calculating work of single learning and the decrease of converging rate.
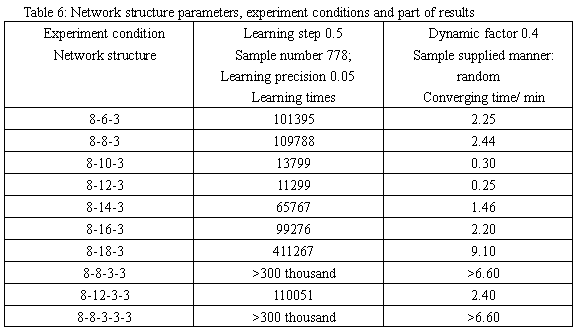
When the number of hidden layer unit ranges in 1.2~1.8 times of input layer unit, model converges well; and when hidden layer unit number increases again, model’s converging property slightly decrease accordingly.
(2) Compared with single hidden layer network, double hidden layer network’s converging rate decreases as learning time increases with the same learning precision and hidden layer unit number.
(3) Under the same condition, triple hidden layer network’s learning time and converging time all sharply increase.
Summarizing all above discussion, this paper selects single hidden layer network, considering network’s strength, the hidden layer unit number is better to be 1.2~1.8 times of input layer unit number.
3.2.4 Other aspects
Due to the non-linear relationship between input and output of feed forward neuron network, setting of initial weight has great influence on whether the learning is located on local minimal point and whether leaning converges. Commonly, every neuron’s status value is expected to approach zero when initial weight is inputted and accumulated, which makes sure that iteration in the flat field of error curved face at the beginning. In practice, weight is initialized with random number, which ranges in [-0.5, 0.5], and convergence performances well.
3.3 Checking forecasting precision of neuron network
In this paper, the model is gained when η=0.5, α=0.4, sample number is 778, learning times is 3.12��105 , learning precision is 0.04. In order to check the forecasting precision of the neuron network model, we use the known chemical compositions and hot-rolling process parameters to forecast the yield strength, tensile strength and percentage extension before a batch of gas cylinder steel is checked. The contrast between forecasting value and practical value are described in table 7(only part of results). The complete forecasting results are described in figure 2~3. The results indicate that this neuron network system’s forecasting precision is basically below 10% and the model can be spread.
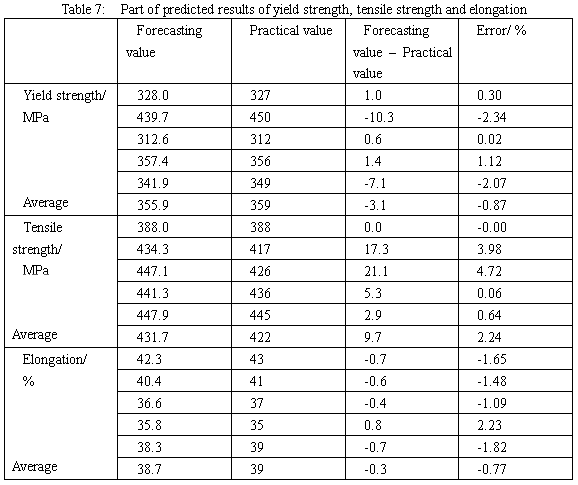
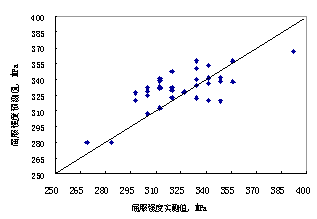
Fig. 2 Predicted precision analysis of yield strength
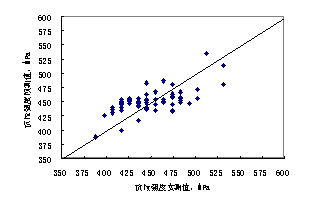
Fig.3 Predicted precision analysis of tensile strength
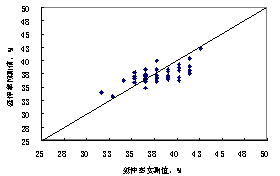
Fig.4 Predicted precision analysis of elongation.
4 Conclusion
(1) Based on feed forward artificial neuron network’s functions of non-linear approaching, fault-tolerant, self-learning, self-organizing and self-adapting, the forecasting model of gas cylinder steel’s mechanical property is built up. We can forecast with high precision the mechanical properties according to the chemical compositions and process parameters. The forecasting precision is below 10%.
(2) Utilizing the ANN forecasting model of gas cylinder steel, we can get more precise rules that rolling process parameters and chemical compositions influence mechanical properties, which benefit the process control.
Reference
1 Chen guoliang. Theory evolvement of artificial neuron network.. Electronic Journal, 1996, (1): 70~75,
2 Cheng xiangjun Principle of neural network and its application.National defence industry press, 1995:24~25, Beijing
3 Yan pingfan Artificial neuron network—model analysis and application Anhui education press, 1993: 13~15, Hefei
4 Zhang liming Model of artificial neuron network and its application Fudan university press, 1993, 13~15, Shanghai
5 Wang tie Effect of the learning rate and shape factor on the learning speed in algorithm Journal of Shanghai JiaoTong University, 1997; (3): 109~112
6 Ai liqun Application of artificial neural network in metallurgical industry Journal of iron and steel research, 1997; (4): 60~63
7 Jiang jianhua Prediction of properties of IF steel using neural network model Journal of university of science and technology Beijing, 1997; (5):467~470
|